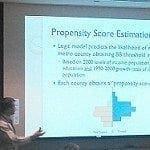
Many studies in public health, including comparative effectiveness research, aim to answer questions such as “what works for whom?” or “under what conditions does it work?” Such questions can often only be answered in the context of a non-experimental study. Propensity scores are a key statistical tool for non-experimental studies because they facilitate the comparison of “apples with apples.” However, many non-experimental studies require combining information from multiple data sets, which may have slightly different measures available. Examples include depression measured using two different scales, or direct- versus parent-reported measures of behavior among children with autism. Existing propensity score methods cannot handle situations where the covariates are measured with error or are measured differently across treatment and comparison groups. This is a particular challenge in mental health research, where many of the disorders and factors under study are not directly observable and are instead modeled as latent constructs. This project will develop and assess new statistical methods for estimating treatment effects in non-experimental studies when the covariates are measured with error or are measured in different ways across the treatment and comparison groups. The work will tie together propensity score methods for estimating treatment effects, latent variable methods, and multiple imputation methods for handling missing data. The aims are: 1) Investigate the implications of measurement error in the covariates when using propensity score methods to estimate treatment effects, 2) Develop and assess propensity score methods for settings where some of the covariates are measured with error or are measured differently in the treatment and comparison groups, and 3) Use the results and methods from Aims 1 and 2 to estimate treatment effects in three studies, each comparing a group receiving the intervention to an external comparison group, and then test the methods by comparing those estimates to the reported treatment effects from randomized trials of the same interventions. The methods will be examined in the context of three studies in mental health evaluating the effectiveness of (1) early intervention for children with autism, (2) perinatal depression prevention, and (3) the use of ginkgo biloba to prevent dementia and Alzheimer’s disease. (from NIH RePORT)
Photo: “Propensity Score” by John S. Quarterman is licensed under CC BY 2.0